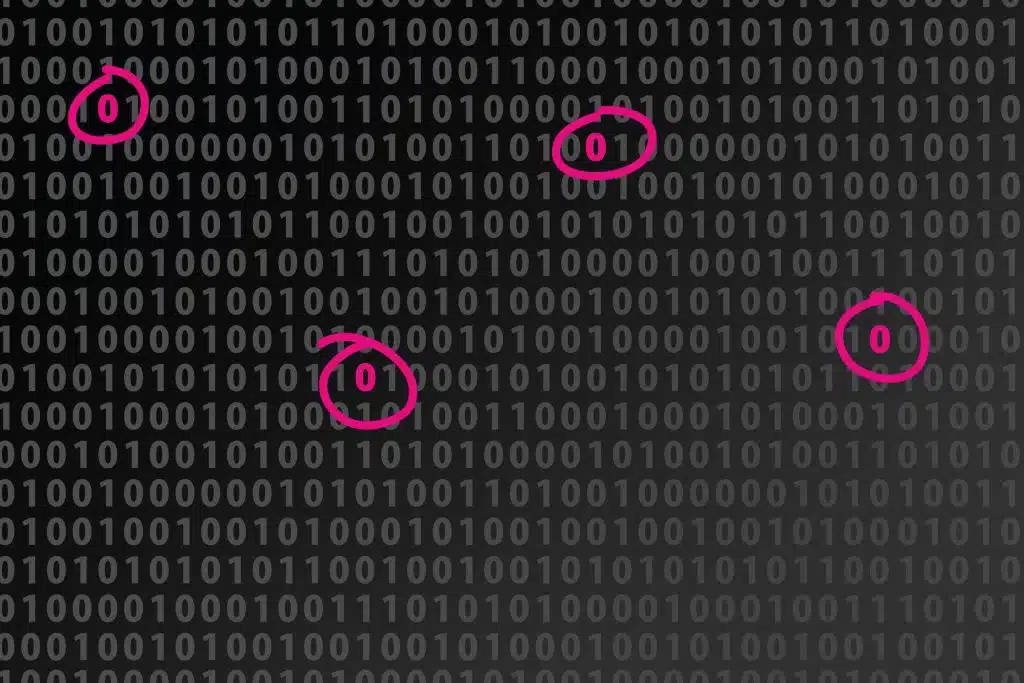
By Mark Montgomery, Strategy & Business Development, big xyt
Enormous volumes of data might be the lifeblood of quantitative analytics, but for the typical trader, dealing with data in any asset class can be complex, costly and daunting. With the explosion of data in recent years and the continuing appearance of new data sources, the challenge for practitioners is growing all the time and they need the power to identify and extract the data that is most relevant to them.
Attempting to isolate data using standard spreadsheets is much like using a bucket and spade to find a grain of sand on a beach – it simply can’t be done. Traders need to understand how data has been sourced and they must be able to curate and store large volumes of data in an accessible and manageable format so that they can easily identify trends as time elapses. Advanced visualisation tools are crucial to enable them to sift through vast amounts of data to capture what is most relevant and use it to enhance their business.
Finding outliers
Identifying outliers should be a routine part of this process. Look at any scatter graph and the outliers are usually obvious enough – the lone dots cast out on a limb, far removed from the core cluster of data points. They usually represent anomalies to a trend, and once investigated, can be explained by a unique change in circumstance.
In the fragmented European equity market with multiple trading venues, outliers are important as they will highlight securities behaving unexpectedly due to a number of possible factors, including poor liquidity, sudden volatility, unreliable data or regulatory changes. Using visualisation techniques to spot and address outliers is fundamental to navigating fragmented liquidity and pursuing best execution in any asset class. The same logic we have applied to equities can be equally applied to fixed income, for example.
When collecting and analysing data from trading venues, outliers should help market participants to validate the data they hold, ensuring it is fit for purpose and identifying any inaccuracies that need to be addressed. Rigorous analysis of outliers can also help firms to ensure they are accessing the best liquidity, and getting the best possible service from their counterparties.
Identifying causes
Addressing the cause of outliers doesn’t always come naturally, particularly when dealing with a large range of complex data sources and having to bring consistency to conflicting taxonomies. Market participants must train themselves not only to be on the look-out for outliers, but also to identify what has caused them.
Users must first think about relevance, establishing whether a particular outlier is really significant and will actually influence behaviour. If it is only a single outlier, or a very small number, it may be just an anomaly that is not worth investigating. More numerous outliers should be addressed, however, to ensure optimum execution.
Once outliers have been determined to be relevant, they can then be used to validate data. If a data set is full of outliers, for example, its accuracy must be questioned. Venues will typically use their own mappings and time stamps. In spite of efforts to standardise these, the presence of outliers may be a sign of inaccurate or inconsistent mappings that needs to be addressed.
Constant scrutiny
Even after a data set is deemed to be accurate and reliable, outlier detection should still be an integral part of the quest for liquidity and best execution. This should enhance the dialogue with counterparties, making sure they perform consistently in line with instructions. An absence of outliers may suggest all is well, but users must continue to scrutinise data from venues and counterparties on an ongoing basis.
In an age when data is becoming ever more important, outliers are an inevitable reality. In any data set, there will always be outliers, but with the right visualisation tools in place, market participants should be able to make better sense of them, using outlier detection to make better trading decisions and improve execution quality.